Características cualitativas del valgo frontal de rodilla durante el aterrizaje en diferentes categorías de la selección femenina de fútbol de Chile a partir de videos 2D: Conocimientos del aprendizaje en conjunto
Qualitative features of frontal knee valgus patterns during landing in different categories of the female chilean national soccer team from 2D Videos: Insights from ensemble learning
Características qualitativas dos padrões de valgo frontal do joelho durante a aterrissagem em diferentes categorias
Carlos De la Fuente,1,*
Roberto Yañez,2,3
Matias Roby,3
Rony Silvestre.3,4
- Faculty of Rehabilitation Sciences, School of Physical Therapy, Exercise and Rehabilitation Sciences Institute, Universidad Andres Bello, Santiago, Chile; (2) Traumatologia, Clinica MEDS, Santiago, Chile. (3) Centro de Innovación, Clinica MEDS, Santiago Chile; (4) Carrera de Kinesiologia, Departamento de Ciencias de la Salud, Facultad de Medicina, Pontificia Universidad Catolica de Chile, Santiago, Chile.
Conflicts of Interest: The authors declare no conflict of interest.
Correspondence:
Dr. C. de la Fuente
Zipcode: 7960000
Tel.: +56-9-54265340
Abstract
Introduction: Frontal knee valgus at landing is associated with knee injuries. Thus, video records may be helpful for injury risk screening. Here, we determine the feature rank importance of knee valgus patterns in the frontal plane during drop jump landing in different Female Chilean national Soccer team categories.
Materials and Methods: Thirty-nine soccer players (aged 19.8 ± 1.1 yrs) were included. Ninety drop jumps were included from 142 frontal videos obtained from the injury surveillance assessments of different categories of the national Chilean team from 2018 to 2020. We create a feature vector from 11 binary features which were studied using random forest classification. 10-folder cross-validation was performed. Features weighted > 0.10 were selected for prediction.
Results: Hip-knee-ankle alignment in bipedal posture, not a parallel inter-patella and inter-ankle straight line with the ground, and patella inward regarding ankle had 0.256, 0.263, 0.147, and 0.208 coefficients, respectively. Cross-validation accuracy was 0.98.
Discussion: The most predictive features are hip-knee-ankle alignment in bipedal posture, not a parallel inter-patella straight line with the ground, not a parallel inter-ankle straight line with the ground, and patella inward regarding the ankle. Furthermore, this study suggests that different female Chilean national soccer team categories develop valgus patterns during landing.
Level of evidence: IIb.
Key-words: Knee, ligament rupture, prevention.
Introduction
The excessive dynamic knee valgus during jumping is considered a mechanism associated with lower extremity injuries [1][2], and a risk population would be female soccer players with suboptimal physical conditioning [3–5]. The dynamic knee valgus results from adduction and medial rotation of the hip, and adduction and lateral rotation of the knee [6]. However, when the dynamic valgus motion is projected in the frontal plane on a two-dimensional video analysis, the frontal plane projection angle (FPPA) is obtained [6]. The FPPA of knee valgus is a valid and reliable measure [6], ranging from 0.59 to 0.98 depending on the two-dimensional method, task analyzed, and type of reliability reported [6]. Their smallest detectable change ranges from 7.54° to 8.93° [1].
The movement behind the FPPA has been qualitatively appreciated as observational screening with high precision but low coherence with FPPA values [7]. This suggests that the qualitative appreciation of movement centers their observation on several different features that allow better performance for knee valgus classification in the frontal plane than only considering a projected angle. These observations have involved the alignment of the knees, at least 1 of the knees moving into a substantial amount of valgus (ie, knee medial to foot) and/or clear mediolateral side-to-side movement of the knee [7]. In addition, FPPA has been qualitatively appreciated through hip-knee-foot alignment, knee inwards position, the parallelism of thighs and foot, or the foot placement shoulder width apart in the tuck jump score [8]. Also, the Landing Error Scoring System looks for motion quality through stance width, foot rotation position, maximum knee-valgus angle, or trunk orientation [9]. These scores have good to excellent reliability for most items, ranging from 0.65 to 0.91 and 0.72 to 0.81, for tuck jump assessment [9] and the Landing Error Scoring System, respectively [9].
Nowadays, the qualitative appreciation of the movement, like FPPA of knee valgus during landing, can be used to solve the classification valgus vs. no valgus movement strategy through feature rank importance [10]. The classification based on multiple features can be helpful in exploring hierarchical relations to determine the valgus classification. Specifically, multiple decision trees based on ensemble learning and bagging to reduce overfitting and obtain controlled variance would be suitable for a final prediction using the Random Forest classifier [11].
Because different categories of the Female Chilean national Soccer team cannot develop the best physical conditioning [4], they would show different FPPA of knee valgus patterns from 2D Videos of drop jumps being relevant to account with different valgus patterns to test qualitative appreciation of the movement by random forest classification. Therefore, we determine the feature rank importance of knee valgus patterns in the frontal plane during drop jump landing in different Female Chilean national Soccer team categories.
Materials and Methods
In this retrospective observational and cross-sectional study, different soccer players from the Female Chilean national soccer team categories performed a drop jump test as a routine screening assessment by the medical team. The same senior sports medicine physical therapists collected all 2D videos in the frontal plane. We binary scored 6 and 5 features in the vertical and horizontal image axis to create a feature vector of knee valgus. The feature vectors of the whole sample were studied using random forest classification to determine feature hierarchical dependences regarding valgus and not valgus prediction. The study was approved by the institutional review board from the Pontifical Catholic University Ethical Committee (Santiago, Chile) and was conducted according to the Helsinki Declaration.
Thirty-nine female Elite soccer players (aged 19.8 ± 1.1 years, height 1.65 ± 0.05 m) were included. From 142 frontal 2D vertical jump videos obtained from the injury surveillance assessments of the National Association of Professional Soccer on the national Chilean team from 2018 to 2020, ninety vertical drop jumps were included.
The inclusion criteria for drop jumps were: i) to step off the box using a 2-foot landing strategy and immediately followed by a maximal vertical jump, ii) soccer players of the National Chilean team, and iii) female athletes. The exclusion criteria were i) failure to perform a vertical jump after the first landing, ii) non-indoor training shoes, iii) non-bipedal initial posture, iv) incomplete video drop jump record, and iii) any other condition that alters the jump performance.
Drop Jump
All participants were familiarized with previous drop jump assessments in previous seasons, had around ten minutes of warm-up, and were allowed up to 3 practice trials before testing [7]. Then, participants stepped off a 30-com height box using a 2-foot landing strategy and immediately performed their maximal vertical jump over solid ground. An invalid jump was considered if any exclusion criteria applied to the jump performance.
2D video records
One digital camera of 60 frames per second of 4k was located at 65 cm of height and 1 meter in front at a sufficient distance where the athlete on the box was acquired entirely. Each digital video was recorded in MP4 extension and saved in the database of the National Association of Professional Soccer medical team.
The same senior physical therapist analyzed the videos based on 6 and 5 features in the vertical and horizontal image axis as a binary score (presence or not presence), see Figure 1. The vertical features were the joint center hip-knee-ankle alignment in bipedal posture, not patella high alignment, no vertical trunk alignment, lower leg not parallel alignment, thigh not parallel alignment, and foot placement not parallel alignment. The horizontal features were not a parallel inter-hip straight line with the ground, not a parallel inter-patella straight line with the ground, not a parallel inter-ankle straight line with the ground, foot placement not shoulder width apart, and patella inward regarding ankle.
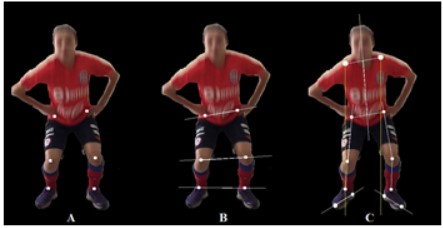
Figure 1. Landmarks and segments observed in the qualitative assessment. A. Qualitative frontal joint of hips (proximal point of tight), patellas (center), and ankles (midpoint between malleolus). B. Inter-hip, -knee, and -ankle straight lines. C. tight (hip-patella segment), leg (patella-ankle segment), foot segments (tip of foot-calcaneus segment), trunk orientation, and shoulder width. The positive patella inward sign is considered when the patella landmark is medial to the ankle.
Data analysis
The first and second landings were used for feature extraction. The valgus or not valgus column vector Y was arranged in the first column, and features X were arranged from columns two until 12 to create a row vector for each drop jump. Then, randomly, the matrix was divided for testing and training matrix in a ratio of 2:8. The hyperparameter max_depth and n_estimator were estimated through grid search. Then, the random forest model classification was performed, and the feature importance based on mean decrease impurity or Gini importance was determined. This is calculated by equations 1, 2, and 3, where the impurity is the Gini index, ni is the –ith feature, Nt is the number of rows that particular node has, N is the total number of rows present in data, Nt (right) is the number of nodes in the right node, Nt (left) is the number of nodes in the left node, and pj proportion of the sample that belongs to class c for a particular node.
Eq.1
Eq.2
Eq.3
The validation of the data analysis using random forest was made by 10 cross-validation folders. Model accuracy and mean decrease impurity with their standard deviation were described. The features with weight > 0.10 were retained for dimension reduction, creating a knee valgus classifier for the whole sample. Thus, the confusion matrix was estimated between the predicted classes using features with weight > 0.10 and all original features. The libraries used for all estimations were Sklearn, Numpy, Pandas, and Matplotlib through Ipython 3.5 (© Copyright the IPython development team, Python Software Foundation) [12].
Results
Sixteen and eighteen valgus occurred during the first and second lading, respectively. Four features had weight > 0.10; the coefficient of hip-knee-ankle alignment in bipedal posture, not a parallel inter-patella straight line with the ground, not a parallel inter-ankle straight line with the ground, and patella inward regarding ankle were 0.256, 0.263, 0.147, and 0.208, respectively (Figure 2). The accuracy of the cross-validation was 0.98.
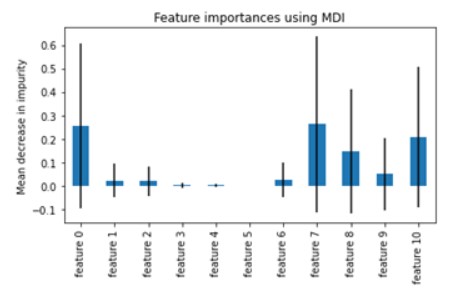
Figure 2. Features relevance obtained after the random forest.
The confusion matrix for the predictive features is summarized in Table 1. The sensitivities were 0.97, 0.82, 0.74, and 1.00 for hip-knee-ankle alignment in bipedal posture, not a parallel inter-patella straight line with the ground, not a parallel inter-ankle straight line with the ground, and patella inward regarding ankle, respectively. The specificities were 0.89, 0.95, 0.91, and 1.00 for hip-knee-ankle alignment in bipedal posture, not a parallel inter-patella straight line with the ground, not a parallel inter-ankle straight line with the ground, and patella inward regarding ankle, respectively. The accuracies were 0.92, 0.90, 0.84, and 1.00 for hip-knee-ankle alignment in bipedal posture, not a parallel inter-patella straight line with the ground, not a parallel inter-ankle straight line with the ground, and patella inward regarding ankle, respectively. The precisions were 0.85, 0.90, 0.83, and 1.00 for hip-knee-ankle alignment in bipedal posture, not a parallel inter-patella straight line with the ground, not a parallel inter-ankle straight line with the ground, and patella inward regarding ankle, respectively.
Table 1. Confusion Matrix for the four most predictive features.
Features |
|
True values |
|
||
Hip-knee-ankle alignment: Valgus No Valgus |
|
Valgus |
|
No Valgus |
|
33 1 |
6 50 |
||||
No parallel patella: Valgus No Valgus |
|
Valgus |
|
No Valgus |
|
|
28 6 |
|
3 53 |
||
Not parallel ankle: Valgus No Valgus |
|
Valgus |
|
No Valgus |
|
|
25 9 |
|
5 51 |
||
Patella inward: Valgus No Valgus |
|
Valgus |
|
No Valgus |
|
|
34 0 |
|
0 56 |
Discussion
Our main findings are that 1) there are qualitative features in the frontal plane that allow the classification of knee valgus patterns, where the most predictive features are hip-knee-ankle alignment in bipedal posture, not a parallel inter-patella straight line with the ground, not a parallel inter-ankle straight line with the ground, and patella inward regarding ankle. 2) The patella inward regarding the ankle is the best predictor of frontal knee valgus patterns. Finally, 3) this study suggests that different female Chilean national soccer team categories develop valgus patterns during drop jump. Our findings are relevant for automatized AI screening tools as first assessment or self-video recording for constant surveillance alerts. Drop-jump video analysis is relevant because it is one of the risk criteria for suffering a knee injury [13].
In our study, the best discriminative descriptor of the pattern was the patella inward regarding the ankle. This feature has been suggested to be a medial knee displacement beyond the foot-thigh line in the frontal plane as the result of adduction and internal rotation of the femur, abduction of the knee, anterior tibial translation, external tibial rotation and ankle eversion [14]. After the random forest desition, the patella inward sign showed high sensitivity and specificity in the frontal plane assessment for drop jump videos. The valgus pattern classification based on qualitative video screening is in accordance with the clinical identification of female athletes with high knee valgus angles in a vertical drop-jump landing using real-time screening [7].
Hip-knee-ankle alignment in bipedal posture, not a parallel inter-patella straight line with the ground, and not a parallel inter-ankle straight line with the ground, had the next higher sensitivity and specificity. This suggests that these features are complementary descriptors of the valgus pattern, which can be useful for knee valgus classification. These features represent a basic geometry of lower limbs, which show dependence regarding contralateral or vertical joint alignment. Likely, these identified asymmetries would be more related to different mechanisms that can be or are not involved with knee valgus pattern exclusively, like trunk or ankle uncontrol [15,16]. Because of that, their sensitivity and specificity would decrease, as suggested have occurred in our research.
Finally, our study suggests that this study suggest that different categories of the female Chilean national soccer team develop valgus patterns during drop jumps. Related studies with athletes have found that most untrained female athletes demonstrated valgus knee patterns, but after neuromuscular training, female athletes can improve the trunk and lower limb alignment during landing and takeoff tasks [17]. The clinical challenge for clinicians and bio-mechanists is to know on time when an athlete starts developing knee valgus patterns during landing tasks. This knowledge would allow us to plan assertive interventions. Thus, our study opens the chance to use feature extraction in the frontal plane of drop jumps as a knee valgus pattern marker for surveillance.
Our study is not out of limitations. In this study, the pose algorithm may have improved the joint centers for monocular pose detection and massive image feature extraction stay in uncertainty to have improved the knee valgus pattern classification. Also, more sophisticated AI algorithms need to be tested in the future. Also, a deep effort would be developed in the future for real-time detection during matches.
There are qualitative features in the frontal plane that allow classifying knee valgus patterns, where the most predictive features are hip-knee-ankle alignment in bipedal posture, not a parallel inter-patella straight line with the ground, not a parallel inter-ankle straight line with the ground, and patella inward regarding ankle. Furthermore, this study suggests that different female Chilean national soccer team categories develop valgus patterns during drop jumps and are at knee injury risk.
References
1. Munro A, Herrington L, Carolan M. Reliability of 2-Dimensional video assessment of frontal-plane dynamic knee valgus during common athletic screening tasks. J Sport Rehabil. 2012; 21:7–11.
2. Nilstad A, Krosshaug T, Mok K-M, Bahr R, Andersen TE. Association between anatomical characteristics, knee laxity, muscle strength, and peak knee valgus during vertical drop-jump landings. J Orthop Sports Phys Ther. 2015; 45:998–1005.
3. Brophy RH, Stepan JG, Silvers HJ, Mandelbaum BR. Defending puts the anterior cruciate ligament at risk during soccer: a gender-based analysis. Sports Health. 2015; 7:244–9.
4. Villaseca-Vicuña R, Jesam-Sarquis F, Mardones C, Moreno C, Peréz-Contreras J. Comparison of physical fitness and anthropometric profiles among chilean female national football teams from U17 to senior categories. JPES 21.
5. Villaseca-Vicuña R, Otero-Saborido FM, Perez-Contreras J, Gonzalez-Jurado JA. Relationship between physical fitness and match performance parameters of chile women’s national football team. Int J Environ Res Public Health. 2021; 18:8412.
6. Scholtes SA, Salsich GB. A dynamic valgus index that combines hip and knee angles: assessment of utility in females with patellofemoral pain. Int J Sports Phys Ther. 2017; 12:333–40.
7. Nilstad A, Andersen TE, Kristianslund E, Bahr R, Myklebust G, Steffen K, et al. Physiotherapists can identify female football players with high knee valgus angles during vertical drop jumps using real-time observational screening. J Orthop Sports Phys Ther. 2014; 44:358–65.
8. Fort-Vanmeerhaeghe A, Montalvo AM, Lloyd RS, Read P, Myer GD. Intra- and Inter-rater reliability of the modified tuck jump assessment. J Sports Sci Med. 2017; 16:117–24.
9. Padua DA, Boling MC, Distefano LJ, Onate JA, Beutler AI, Marshall SW. Reliability of the landing error scoring system-real time, a clinical assessment tool of jump-landing biomechanics. J Sport Rehabil. 2011; 20:145–56.
10. Pedregosa F, Varoquaux G, Gramfort A, Michel V, Thirion V, Grisel O, et al. Scikit-Learn: machine learning in python. J Machine Learn Res. 2011; 12.
11. Fawagreh K, Gaber MM, Elyan E. Random Forests: from early developments to recent advancements. Syst Sci & Cont Eng. 2014; 2:602–9.
12. Perez F, Granger BE. IPython: A system for interactive scientific computing. Comput Sci&Eng. 2007; 9:21–9.
13. Barber-Westin SD, Noyes FR. Objective criteria for return to athletics after anterior cruciate ligament reconstruction and subsequent reinjury rates: A systematic review. Phys Sportsmed. 2011; 39:100–10.
14. Wilczynski B, Zorena K, Slezak D. Dynamic knee valgus in single-leg movement tasks. potentially modifiable factors and exercise training options. A literature review. Int J Environ Res Public Health. 2020; 17:8208.
15. Hewett TE, Myer GD. The mechanistic connection between the trunk, hip, knee, and anterior cruciate ligament injury. Exerc Sport Sci Rev. 2011; 39:161–6.
16. Zazulak BT, Hewett TE, Reeves NP, Goldberg B, Cholewicki J. Deficits in neuromuscular control of the trunk predict knee injury risk: a prospective biomechanical-epidemiologic study. Am J Sports Med. 2007; 35:1123–30.
17. Noyes FR, Barber-Westin SD, Fleckenstein C, Walsh C, West J. The drop-jump screening test: difference in lower limb control by gender and effect of neuromuscular training in female athletes. Am J Sports Med. 2005; 33:197–207.